6 High-level machine intelligence
6.1 The public predicts a 54% likelihood of high-level machine intelligence within 10 years
Respondents were asked to forecast when high-level machine intelligence will be developed. High-level machine intelligence was defined as the following:
We have high-level machine intelligence when machines are able to perform almost all tasks that are economically relevant today better than the median human (today) at each task. These tasks include asking subtle common-sense questions such as those that travel agents would ask. For the following questions, you should ignore tasks that are legally or culturally restricted to humans, such as serving on a jury.12
Respondents were asked to predict the probability that high-level machine intelligence will be built in 10, 20, and 50 years.
We present our survey results in two ways. First, we show the summary statistics in a simple table. Next, to compare the public’s forecasts with forecasts made by AI researchers in 2016 (Grace et al. 2018), we aggregated the respondents’ forecasts using the same method. Note that Grace et al. (2018) gave a stricter definition of high-level machine intelligence that involved machines being better than all humans at all tasks.13
Year | Respondent type | 25th percentile | Median | Mean | 75th percentile | N |
---|---|---|---|---|---|---|
2028 | All respondents | 30% | 54% | 54% | 70% | 2000 |
2038 | All respondents | 50% | 70% | 70% | 88% | 2000 |
2068 | All respondents | 70% | 88% | 80% | 97% | 2000 |
2028 | No CS or engineering degree | 30% | 54% | 55% | 70% | 1805 |
2038 | No CS or engineering degree | 50% | 70% | 71% | 88% | 1805 |
2068 | No CS or engineering degree | 70% | 88% | 81% | 98% | 1805 |
2028 | CS or engineering degree | 30% | 50% | 48% | 70% | 195 |
2038 | CS or engineering degree | 50% | 70% | 67% | 88% | 195 |
2068 | CS or engineering degree | 50% | 73% | 69% | 97% | 195 |
Respondents predict that high-level machine intelligence will arrive fairly quickly. The median respondent predicts a likelihood of 54% by 2028, a likelihood of 70% by 2038, and a likelihood of 88% by 2068, according to Table 6.1.
These predictions are considerably sooner than the predictions by experts in two previous surveys. In Müller and Bostrom (2014), expert respondents predict a 50% probability of high-level human intelligence being developed by 2040-2050 and 90% by 2075. In Grace et al. (2018), experts predict that there is a 50% chance that high-level machine intelligence will be built by 2061. Plotting the public’s forecast with the expert forecast from Grace et al. (2018), we see that the public predicts high-level machine intelligence arriving much sooner than experts forecast. Employing the same aggregation method used in Grace et al. (2018), Americans predict that there is a 50% chance that high-level machine intelligence will be developed by 2026.
Results in Walsh (2018) also show that the non-experts (i.e., readers of a news article about AI) are more optimistic in their predictions of high-level machine intelligence compared with experts. In Walsh’s study, the median AI expert predicted a 50% probability of high-level machine intelligence by 2061 while the median non-expert predicted a 50% probability by 2039. In our survey, respondents with CS or engineering degrees, compared with those who do not, provide a somewhat longer timeline for the arrival of high-level machine intelligence, according to Table 6.1. Nevertheless, those with CS or engineering degrees in our sample provide forecasts are more optimistic than those made by experts from Grace et al. (2018); furthermore, their forecasts show considerable overlap with the overall public forecast (see Figure 6.1).
The above differences could be due to different definitions of high-level machine intelligence presented to respondents. However, we suspect that it is not the case for the following reasons. (1) These differences in timelines are larger, more significant than we think could be reasonably attributed to beliefs about these different levels of intelligence. (2) We found similar results using the definition in Grace et al. (2018), on a (different) sample of the American public. In a pilot survey conducted on Mechanical Turk during July 13-14, 2017, we asked American respondents about human-level AI, defined as the following:
Human-level artificial intelligence (human-level AI) refers to computer systems that can operate with the intelligence of an average human being. These programs can complete tasks or make decisions as successfully as the average human can.
In this pilot study, respondents also provided forecasts that are more optimistic than the projections by AI experts. The respondents predict a median probability of 44% by 2027, a median probability of 62% by 2037, and a median probability of 83% by 2067.
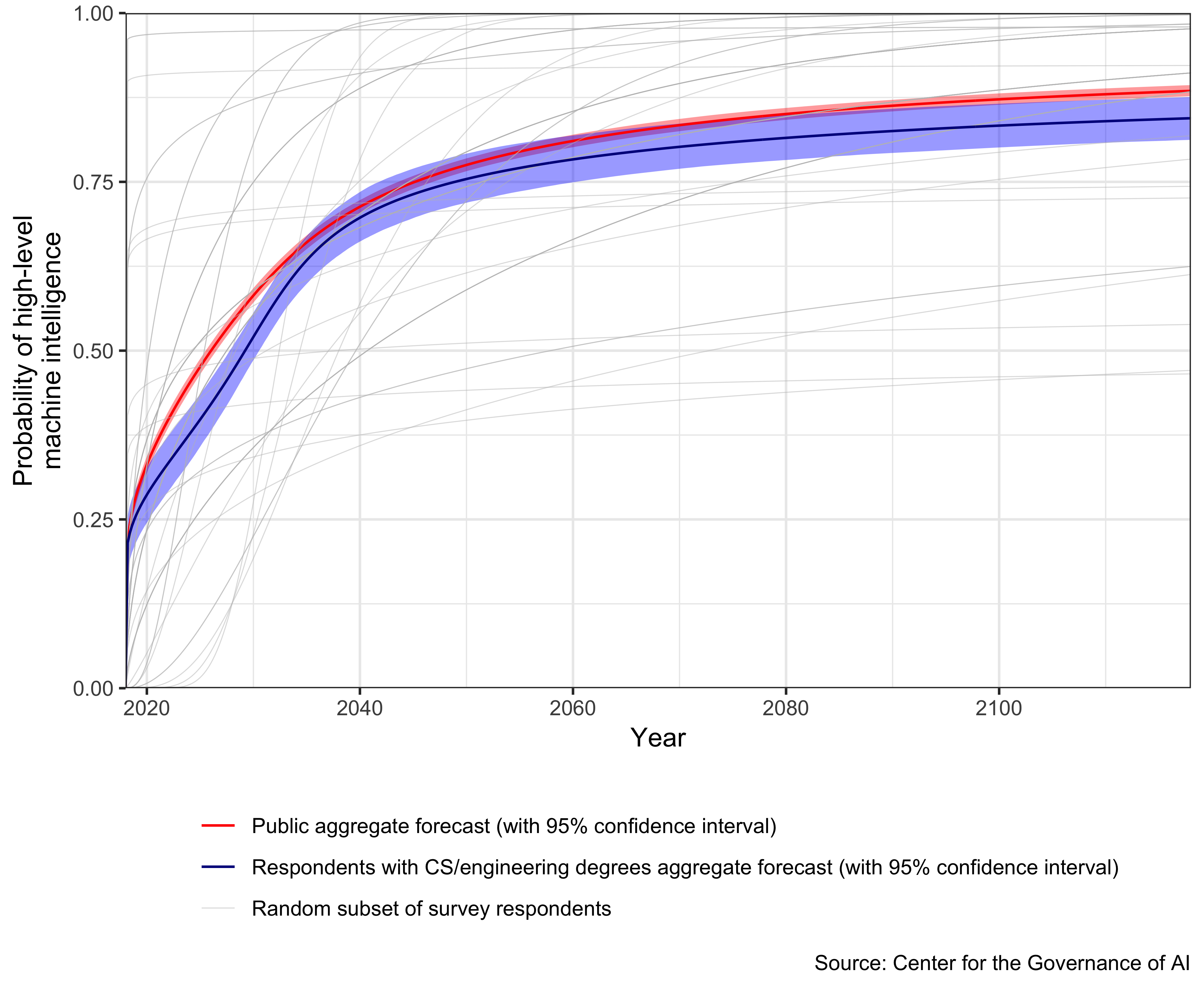
Figure 6.1: The American public’s forecasts of high-level machine intelligence timelines
6.2 Americans express mixed support for developing high-level machine intelligence
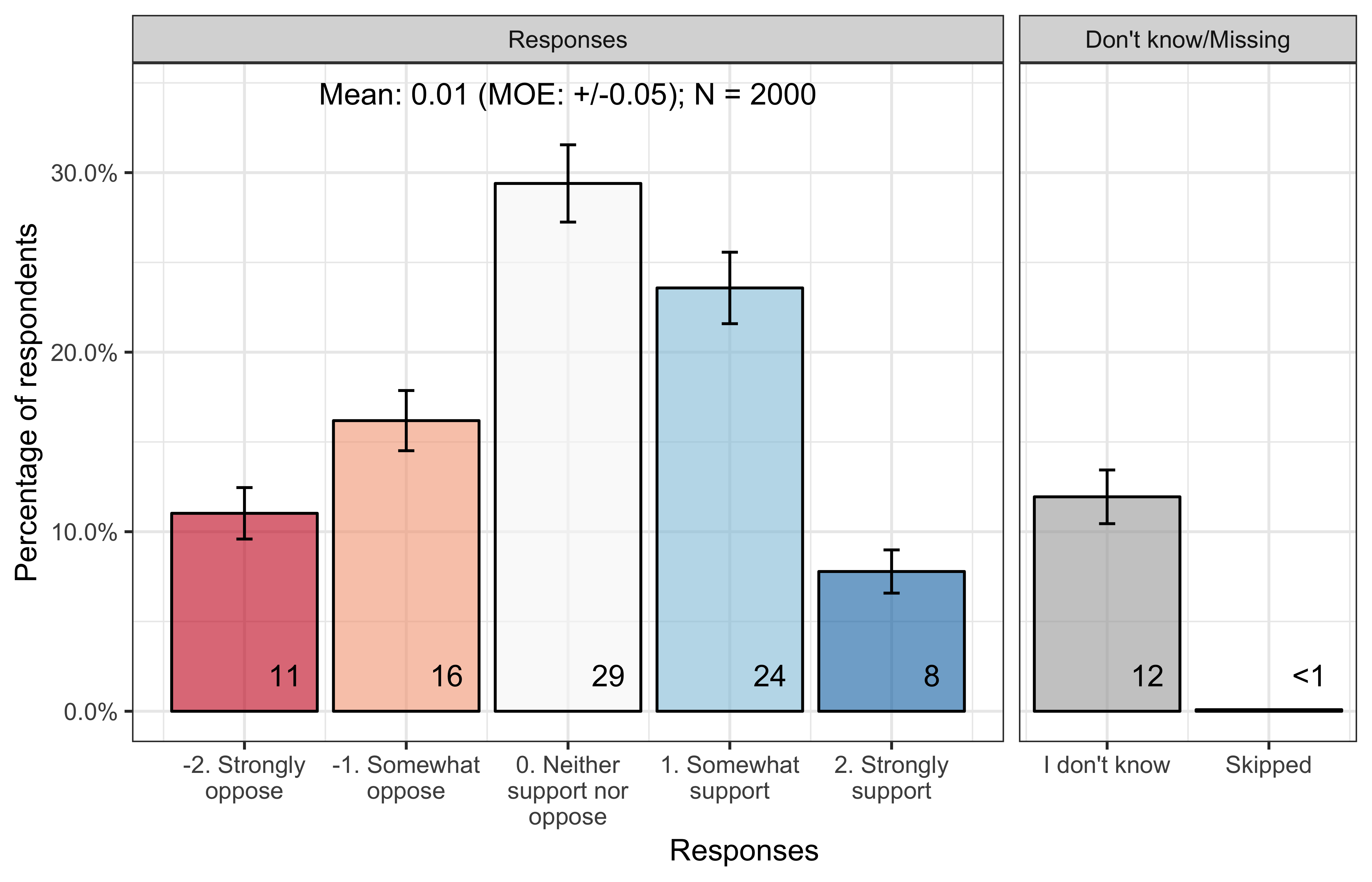
Figure 6.2: Support for developing high-level machine intelligence
Respondents were asked how much they support or oppose the development of high-level machine intelligence. (See Appendix B for the question text.) Americans express mixed support for developing high-level machine intelligence, much like how they feel about developing AI. About one-third of Americans (31%) somewhat or strongly support the development of high-level machine intelligence, while 27% somewhat or strongly oppose it.14 Many express a neutral attitude: 29% state that they neither support nor oppose, while 12% indicate they don’t know.
The correlation between support for developing AI and support for developing high-level machine intelligence is 0.61. The mean level of support for developing high-level machine intelligence, compared with the mean level of support for developing AI, is 0.24 points (MOE = +/- 0.04) lower on a five-point scale (two-sided \(p\)-value \(<0.001\)), according to Table C.31.
6.3 High-income Americans, men, and those with tech experience express greater support for high-level machine intelligence
Support for developing high-level machine intelligence varies greatly between demographic subgroups, although only a minority in each subgroup supports developing the technology. Some of the demographic trends we observe regarding support for developing AI also are evident regarding support for high-level machine intelligence. Men (compared with women), high-income Americans (compared with low-income Americans), and those with tech experience (compared with those without) express greater support for high-level machine intelligence.
We used a multiple regression that includes all of the demographic variables to predict support for developing high-level machine intelligence. The support for developing AI outcome variable was standardized, so it has mean 0 and unit variance.
Significant predictors correlated with support for developing high-level machine intelligence include:
- Being male (versus being female)
- Identifying as a Republican (versus identifying as an Independent or “other”)15
- Having a family income of more than $100,000 annually (versus having a family income of less than $30,000 annually)
- Having CS or programming experience (versus not having such experience)
This last result about women less supportive of developing high-level machine intelligence than men is noteworthy as it speaks to the contrary claim sometimes made that it is primarily men who are concerned about the risks from advanced AI. Men are argued to be disproportionately worried about human-level AI because of reasons related to evolutionary psychology (Pinker 2018) or because they have the privilege of not confronting the other harms from AI, such as biased algorithms (Crawford 2016).
We also performed the analysis above but controlling for respondents’ support for developing AI (see Appendix). Doing so allows us to identify subgroups those attitudes toward AI diverges from their attitudes toward high-level machine intelligence. In this secondary analysis, we find that being 73 or older is a significant predictor of support for developing high-level machine intelligence. In contrast, having a four-year college degree is a significant predictor of opposition to developing high-level machine intelligence. These are interesting inversions of the bivariate association, where older and less educated respondents were more concerned about AI; future work could explore this nuance.
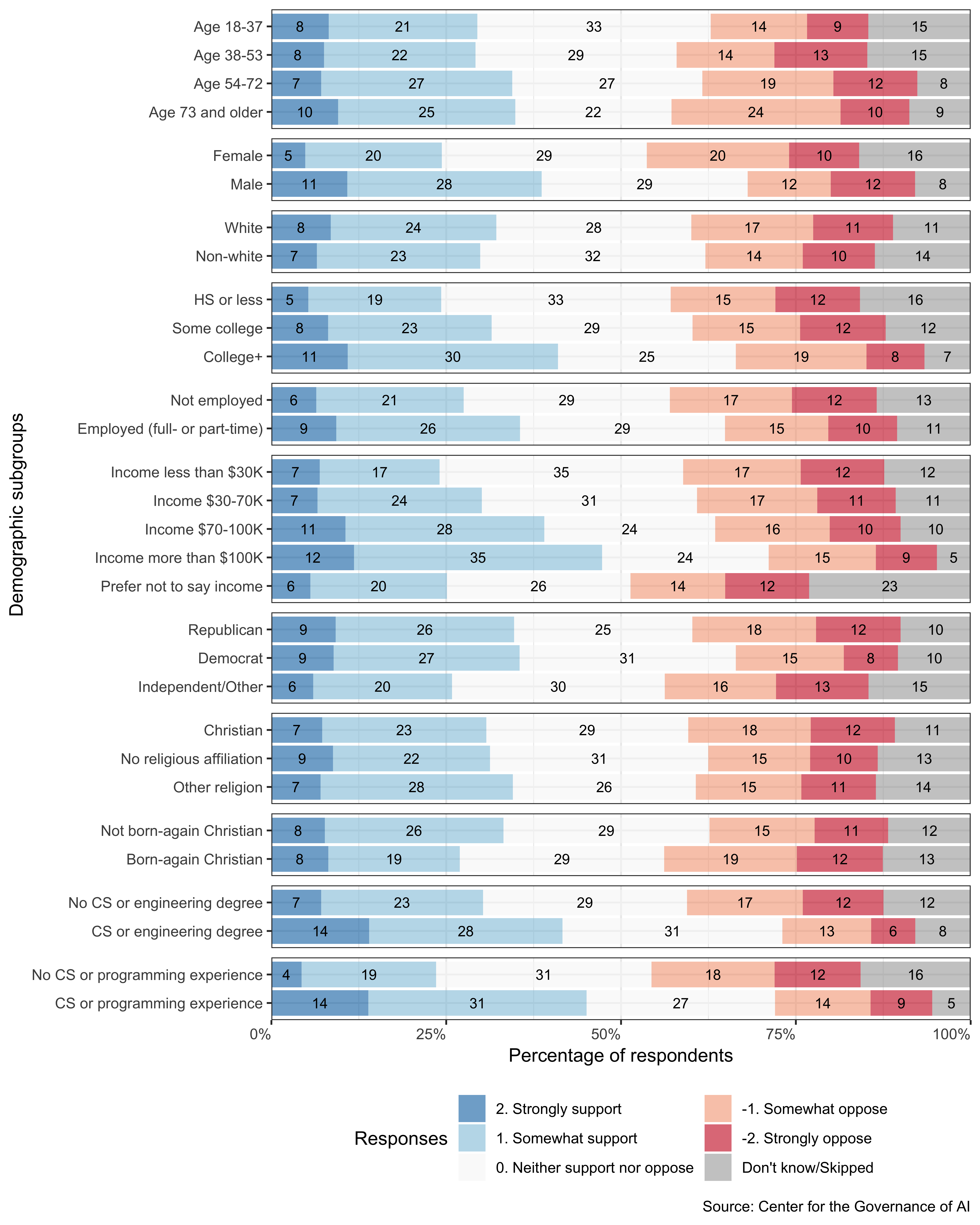
Figure 6.3: Support for developing high-level machine intelligence across demographic characteristics: distribution of responses
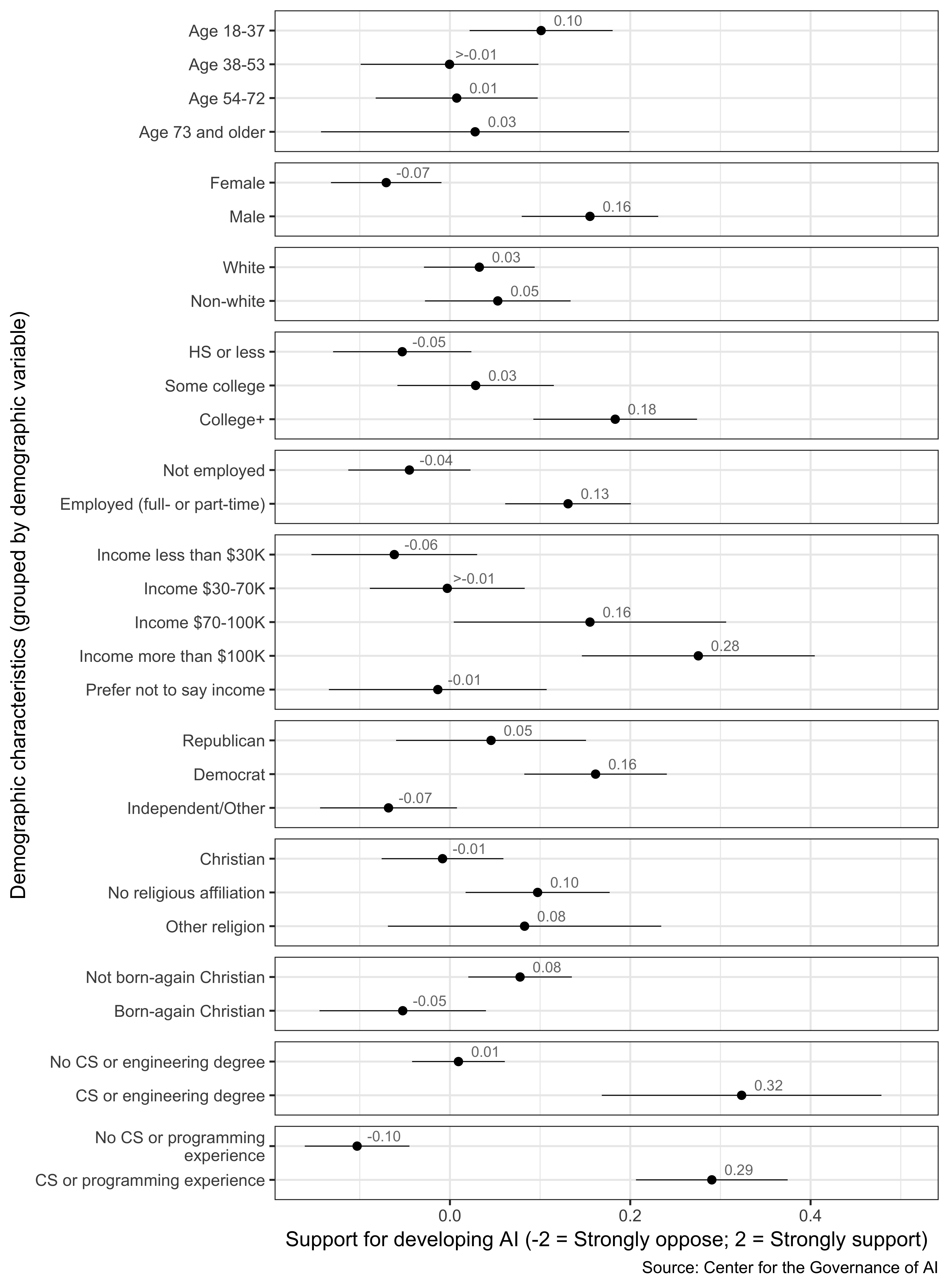
Figure 6.4: Support for developing high-level machine intelligence across demographic characteristics: average support across groups
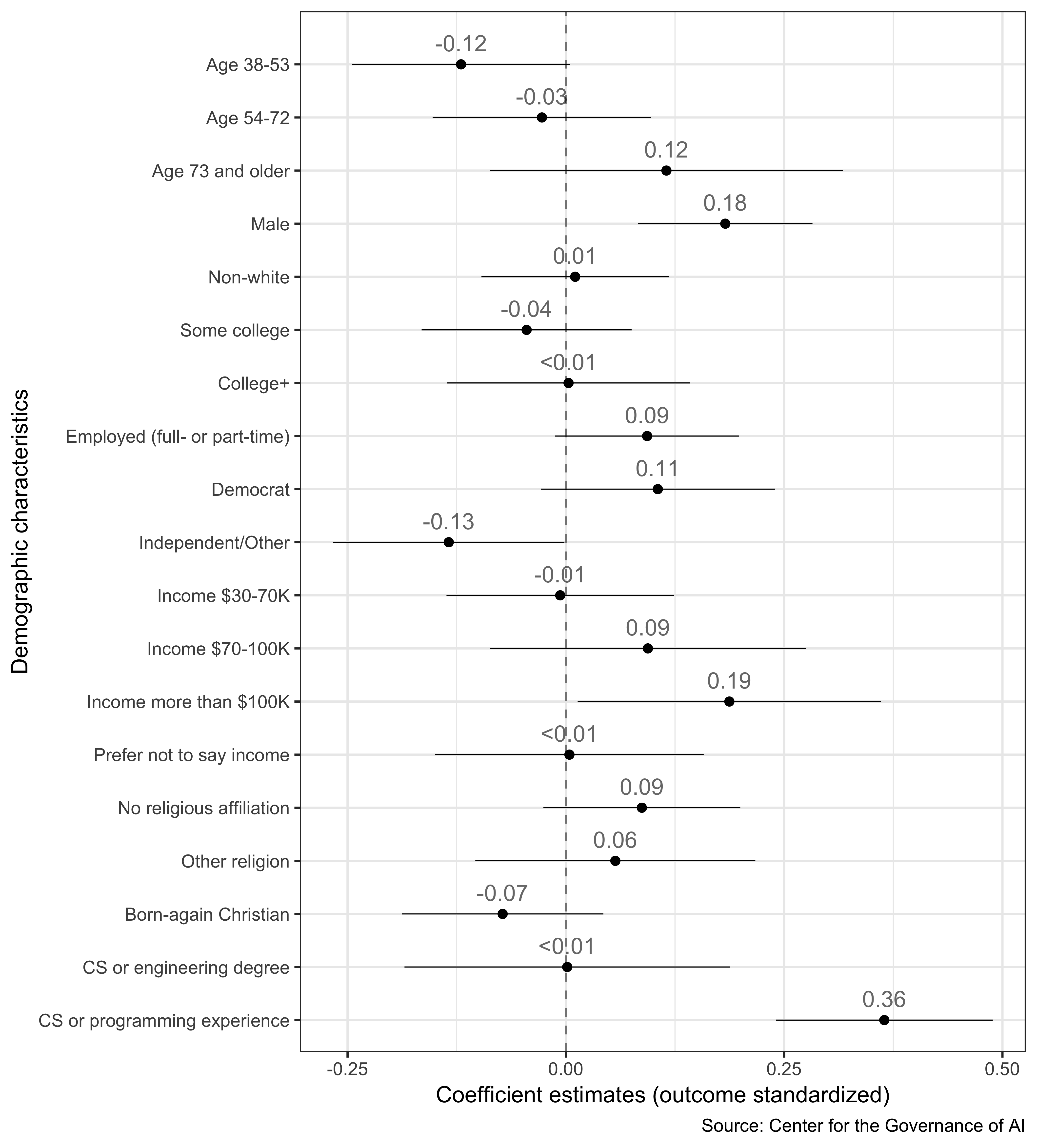
Figure 6.5: Predicting support for developing high-level machine intelligence using demographic characteristics: results from a multiple linear regression that includes all demographic variables
6.4 The public expects high-level machine intelligence to be more harmful than good
This question sought to quantify respondents’ expected outcome of high-level machine intelligence. (See Appendix B for the question text.) Respondents were asked to consider the following:
Suppose that high-level machine intelligence could be developed one day. How positive or negative do you expect the overall impact of high-level machine intelligence to be on humanity in the long run?
Americans, on average, expect that high-level machine intelligence will have a harmful impact on balance. Overall, 34% think that the technology will have a harmful impact; in particular, 12% said it could be extremely bad, leading to possible human extinction. More than a quarter of Americans think that high-level machine intelligence will be good for humanity, with 5% saying it will be extremely good. Since forecasting the impact of such technology on humanity is highly uncertain, 18% of respondents selected “I don’t know.” The correlation between one’s expected outcome and one’s support for developing high-level machine intelligence is 0.69.
A similar question was asked to AI experts in Grace et al. (2018); instead of merely selecting one expected outcome, the AI experts were asked to predict the likelihood of each outcome. In contrast to the general public, the expert respondents think that high-level machine intelligence will be more beneficial than harmful.16 Although they assign, on average, a 27% probability of high-level machine intelligence of being extremely good for humanity, they also assign, on average, a 9% probability of the technology being extremely bad, including possibly causing human extinction.
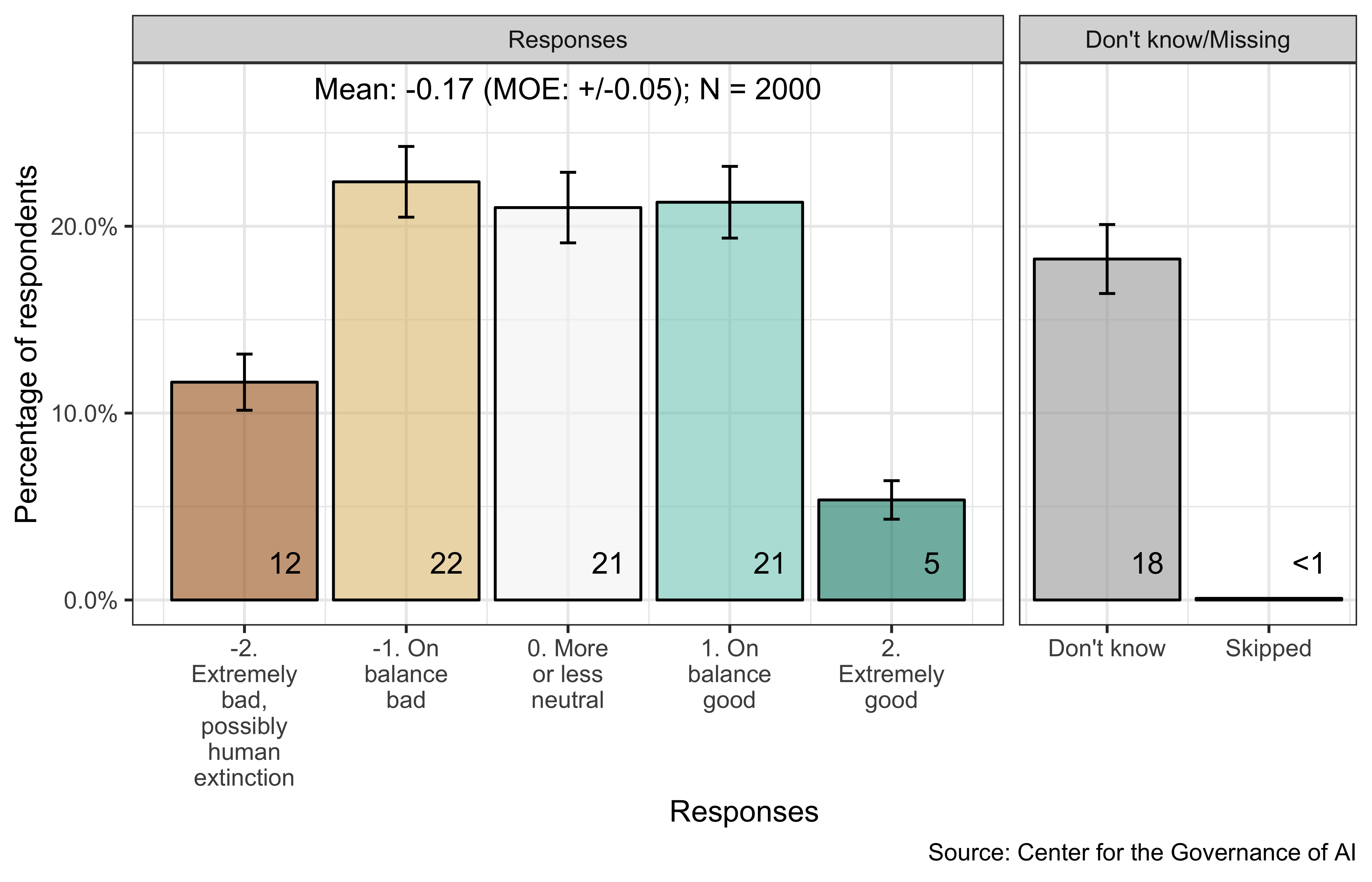
Figure 6.6: Expected positive or negative impact of high-level machine intelligence on humanity
References
Crawford, Kate. 2016. “Artificial Intelligence’s White Guy Problem.” New York Times. https://perma.cc/6C9S-5FNP.
Grace, Katja, John Salvatier, Allan Dafoe, Baobao Zhang, and Owain Evans. 2018. “Viewpoint: When Will AI Exceed Human Performance? Evidence from AI Experts.” Journal of Artificial Intelligence Research 62: 729–54. https://doi.org/10.1613/jair.1.11222.
Müller, Vincent C, and Nick Bostrom. 2014. “Future Progress in Artificial Intelligence: A Poll Among Experts.” AI Matters 1 (1). ACM: 9–11. https://perma.cc/ZY22-E5FF.
Pinker, Steven. 2018. Enlightenment Now: The Case for Reason, Science, Humanism, and Progress. New York: Penguin.
Walsh, Toby. 2018. “Expert and Non-Expert Opinion About Technological Unemployment.” International Journal of Automation and Computing 15 (5): 637–42. https://doi.org/10.1007/s11633-018-1127-x.
Note that our definition of high-level machine intelligence is equivalent to what many would consider human-level machine intelligence. Details of the question are found in Appendix B.↩
In Grace et al. (2018), each respondent provides three data points for their forecast, and these are fitted to the Gamma CDF by least squares to produce the individual cumulative distribution function (CDFs). Each “aggregate forecast” is the mean distribution over all individual CDFs (also called the “mixture” distribution). The confidence interval is generated by bootstrapping (clustering on respondents) and plotting the 95% interval for estimated probabilities at each year. Survey weights are not used in this analysis due to problems incorporating survey weights into the bootstrap.↩
The discrepancy between this figure and the percentages in Figure 6.2 is due to rounding. According to Table B.129, 7.78% strongly support and 23.58% somewhat support; therefore, 31.36% – rounding to 31% – of respondents either support or somewhat support.↩
In the survey, we allowed those who did not identify as Republican, Democrat, or Independent to select “other.” The difference in responses between Republicans and Democrats is not statistically significant at the 5% level. Nevertheless, we caution against over-interpreting these results related to respondents’ political identification because the estimated differences are substantively small while the correlating confidence intervals are wide.↩
To make the two groups’ results more comparable, we calculated the expected value of the experts’ predicted outcomes so that it is on the same -2 to 2 scale as the public’s responses. To calculate this expected value, we averaged the sums of each expert’s predicted likelihoods multiplied by the corresponding outcomes; we used the same numerical outcome as described in the previous subsection. The expected value of the experts’ predicted outcomes is 0.08, contrasted with the public’s average response of -0.17.↩